Understanding Machine Learning’s Role in Digital Wallet Fraud Prevention
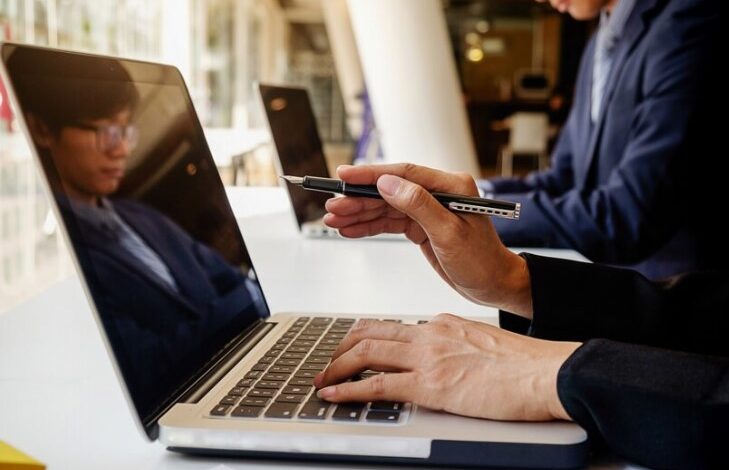
In the digital age, the security of financial transactions is a paramount concern, particularly for users involved in crypto prop trading. Digital wallets have revolutionized the way we handle money, offering unprecedented convenience and accessibility. However, with these advantages come increased risks, including potential fraud and cyber-attacks. Machine learning (ML) has emerged as a critical technology in enhancing fraud prevention for digital wallets, providing advanced methods to detect and mitigate fraudulent activities. This blog explores how machine learning plays a pivotal role in safeguarding digital wallet transactions.
Advanced Fraud Detection
Machine learning algorithms excel at analyzing large volumes of data and identifying patterns that might indicate fraudulent behavior. Traditional fraud detection methods often rely on predefined rules and heuristics, which can be ineffective against sophisticated and evolving fraud techniques. ML, on the other hand, leverages historical data to train models that can detect anomalies and suspicious activities with high accuracy.
For digital wallets used in crypto prop trading, where transactions occur rapidly and in large volumes, ML can monitor transactions in real-time, identifying unusual patterns that may suggest fraudulent activity. For instance, ML models can detect irregular transaction amounts, unexpected changes in transaction frequency, or deviations from typical user behavior, all of which can trigger alerts and preventive measures.
Behavioral Analytics
Behavioral analytics is a powerful ML application that focuses on understanding user behavior and identifying deviations from normal patterns. By continuously analyzing user interactions with their digital wallet, machine learning algorithms can establish a baseline of typical behavior and detect any anomalies that may indicate fraudulent activity.
In the context of crypto prop trading, this means that machine learning can monitor traders’ activities to identify deviations such as unusual trading volumes, abnormal access patterns, or atypical account logins. By leveraging behavioral analytics, digital wallets can enhance their fraud prevention strategies and provide a more secure environment for users engaged in high-stakes trading.
Real-Time Risk Assessment
One of the key advantages of machine learning in fraud prevention is its ability to perform real-time risk assessments. ML algorithms can analyze transaction data as it occurs, assessing the risk level associated with each transaction and providing immediate feedback to users and security systems.
For digital wallet users involved in crypto prop trading, real-time risk assessment is crucial for preventing fraud and ensuring the integrity of transactions. Machine learning models can evaluate factors such as transaction amount, recipient information, and user history to determine the likelihood of fraud. If a transaction is flagged as high risk, the system can prompt additional authentication steps or block the transaction until further verification is completed.
Adaptive Learning
Fraud tactics are constantly evolving, making it essential for fraud prevention systems to adapt and respond to new threats. Machine learning algorithms are capable of adaptive learning, meaning they can continually update their models based on new data and emerging fraud patterns.
In the world of crypto prop trading, where fraudsters are always seeking new methods to exploit vulnerabilities, adaptive learning allows digital wallets to stay ahead of potential threats. By incorporating feedback and new information into their models, ML systems can enhance their detection capabilities and provide more robust protection against sophisticated fraud schemes.
Integration with Other Security Measures
Machine learning does not operate in isolation; it often works in conjunction with other security measures to provide comprehensive fraud prevention. For digital wallets, integrating ML with additional security technologies such as multi-factor authentication (MFA), encryption, and secure access protocols can create a layered defense against fraud.
For users engaged in crypto prop trading, combining ML with these security measures can enhance overall protection. For example, if a machine learning model detects a potential fraud risk, it can trigger additional security checks such as MFA or encryption protocols, adding an extra layer of security to the transaction.
Conclusion
Machine learning is revolutionizing the way digital wallets approach fraud prevention, offering advanced techniques for detecting and mitigating fraudulent activities. By leveraging ML’s capabilities in advanced fraud detection, behavioral analytics, real-time risk assessment, adaptive learning, and integration with other security measures, digital wallets can provide enhanced protection for users involved in crypto prop trading. As fraud tactics continue to evolve, machine learning will play an increasingly critical role in safeguarding digital transactions and ensuring the security and integrity of digital wallet systems.